As social networks are increasingly relied upon to engage with people worldwide, it is crucial to understand and counter fraudulent activities. One of these is “like farming” – the process of artificially inflating the number of Facebook page likes. To counter them, researchers worldwide have designed detection algorithms to distinguish between genuine likes and artificial ones generated by farm-controlled accounts. However, it turns out that more sophisticated farms can often evade detection tools, including those deployed by Facebook.
What is Like Farming?
Facebook pages allow their owners to publicize products and events and in general to get in touch with customers and fans. They can also promote them via targeted ads – in fact, more than 40 million small businesses reportedly have active pages, and almost 2 million of them use Facebook’s advertising platform.
At the same time, as the number of likes attracted by a Facebook page is considered a measure of its popularity, an ecosystem of so-called “like farms” has emerged that inflate the number of page likes. Farms typically do so either to later sell these pages to scammers at an increased resale/marketing value or as a paid service to page owners. Costs for like farms’ services are quite volatile, but they typically range between $10 and $100 per 100 likes, also depending on whether one wants to target specific regions — e.g., likes from US users are usually more expensive.
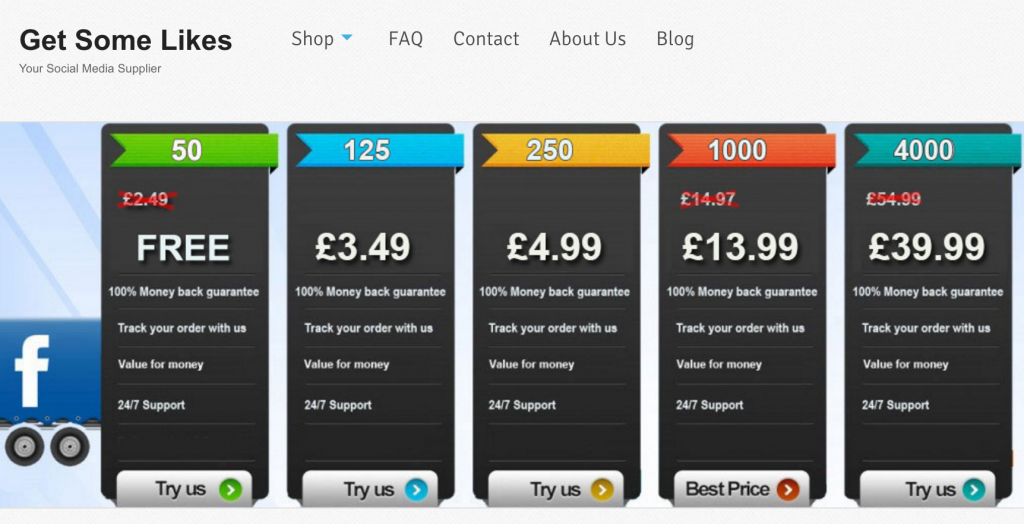
How do farms operate?
There are a number of possible way farms can operate, and ultimately this dramatically influences not only their cost but also how hard it is to detect them. One obvious way is to instruct fake accounts, however, opening a fake account is somewhat cumbersome, since Facebook now requires users to solve a CAPTCHA and/or enter a code received via SMS. Another strategy is to rely on compromised accounts, i.e., by controlling real accounts whose credentials have been illegally obtained from password leaks or through malware. For instance, fraudsters could obtain Facebook passwords through a malicious browser extension on the victim’s computer, by hijacking a Facebook app, via social engineering attacks, or finding credentials leaked from other websites (and dumped on underground forums) that are also valid on Facebook.
Like farms’ operators do not necessarily have to compromise or create fake accounts themselves, but they can also buy some off black markets. However, it is far from the truth to assume that fake likes are only generated by fake/compromised accounts (“bots”), as some of them actually rely on collusion networks where “real” users are incentivized to deliver likes from their accounts. These networks may employ people in exchange of other services or micro-payments. There are also ways to lure real users to like a page – e.g., by promising them access to lotteries, discounts, censored content, etc.
Countering Like Farming
The way fake likes are generated is directly responsible for how difficult it is to counter farms, as well as how they negatively impact legitimate businesses. In our 2014 empirical study, “Paying for Likes? Understanding Facebook Like Fraud Using Honeypots”, we observed two main “modi operandi”: a first set of farms did not really try to hide the nature of their operations, delivering likes in bursts and forming very disconnected social sub-graphs, while another group followed a stealthier approach, mimicking regular users’ behavior and relying on a large and well-connected network structure to gradually deliver likes, while keeping a small count of likes per user.
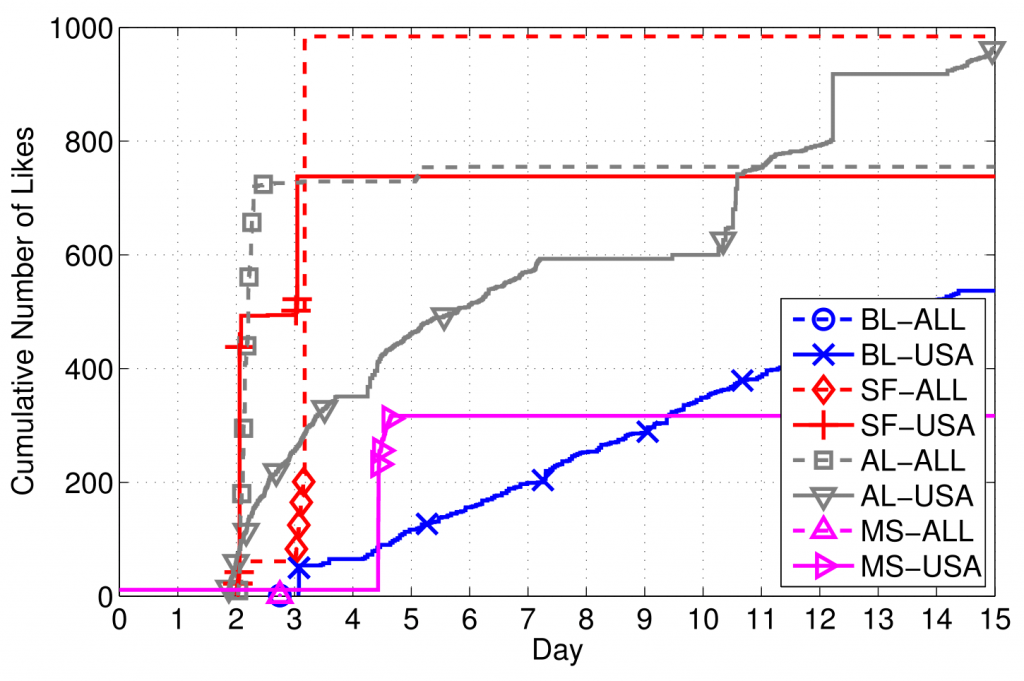
Besides allowing users to artificially manipulate the reputation of their page, like farming also causes a number of non-genuine likes to go toward “unaware” pages. Aiming to hide their activities, accounts operated by farms like popular pages as well as pages actually advertised through Facebook. While the former can at most cause embarrassment (as in the case of Hillary Clinton, whose Facebook account suddenly received thousands of likes from Thailand and Myanmar overnight), the latter actually harms legitimate Facebook users who run paid advertising campaigns. Arguably, these do so aiming to have new users engage with them, but like farm accounts ostensibly do not.
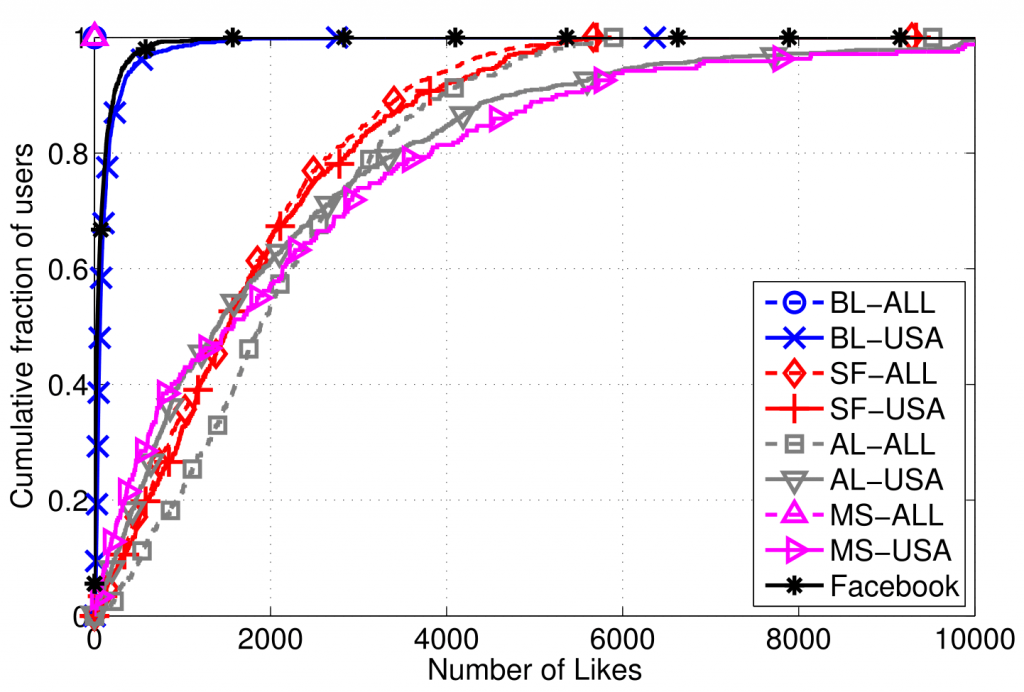
Aiming to counter the farming phenomenon, researchers worldwide, as well as, obviously, Facebook, have been working on computer algorithms that can counter reputation manipulation. In particular, Facebook — in collaboration with university researchers — has developed and deployed several tools to detect spam and fake likes. One, called CopyCatch, detects “lockstep” like patterns by analyzing the social graph between the users and the pages, and the times at which the edges in the graph are created. Another one, called SynchroTrap, relies on the fact that malicious accounts usually perform loosely synchronized actions in a variety of social network context, and can cluster (thus, detect) malicious accounts that act similarly at around the same time for a sustained period of time.
The issue with these methods, however, is that stealthier (and more expensive) like farms — which likely do not rely on fake/compromised accounts — can successfully circumvent them, as a result of spreading likes over longer timespans and liking popular pages to mimic normal users. Our recent preliminary study confirms this hypothesis on accounts used by BoostLikes.com, showing that tools similar to those deployed by Facebook (which rely on graph co-clustering) fail to accurately detect fraud.
Like farm detection tools has thus far almost exclusively focused on activity patterns of pages and users, which fails to capture important characteristics of real accounts employed by the farms. In our recent study, we set to address this gap by looking at timeline features, i.e., how and what users post on Facebook, in order to improve the accuracy of detection mechanisms. We find that posts made by like farm accounts have fewer words, a more limited vocabulary, and lower readability than normal users’ posts. Moreover, their posts are highly targeted to some specific topics, generate significantly more comments and likes, and a large fraction of their posts consists of non-original and often redundant “shared activity” (i.e., repeatedly sharing posts made by other users, articles, videos, and external URLs). Therefore, based on these timeline-based features, we trained machine learning classifiers and evaluated their accuracy on accounts previously gathered from like farm campaigns, achieving near-perfect accuracy also on stealthy farms such as BoostLikes.com.
Naturally, the jury is still out on the effectiveness and the scalability of deploying algorithms that use timeline features for fraud detection over billions of posts. It is also interesting to understand how costly it will be for malevolent actors to change their posting behavior in order to mimic “innocent” users and evade detection. As often happens, fraud mitigation is an economic cat-and-mouse game.
A shorter version of this article was originally published on The Conversation, written by Emiliano De Cristofaro, UCL.
You did a study on this and don’t know that other peoples credentials can’t easily be used? Facebook puts up a, ‘roadblock’.
Also, how exactly does an account, ‘liking’ a page, “negatively impact legitimate businesses” ? OH no we’re suddently very popular… ???
If you are paying Facebook to advertise your page you probably want to attract users who may be interested, and likely to engage with, your page. But if the ads are clicked by farm accounts, then the likelihood of these accounts engaging with your page is close to zero.